Don't tell me what you think. Tell me what is in your portfolio - Nassim Taleb
- Adaptive Alph
- Jan 19, 2020
- 9 min read
Updated: Jan 21, 2020
Black Box:
According to the 2018 Preqin Global Hedge Fund Report, total AUM for hedge funds have now surpassed USD 3.2 trillion. Out of all these hedge funds, the top five largest, ranked by AUM, were all categorized as quantitative investment firms by the well regarded publisher, Pension and Investment. Despite all assets managed by quants, there are plenty of investors and people in general feeling that so called quant strategies are hidden, secret and complex black boxes making unexplainable decisions in the financial markets. Adaptive Alph is here to tell you that the quantitative investment approach or portfolio construction is not a black box. In fact, a true quant approach should be the complete opposite, as really successful quantitative investors must follow the same scientific approach as Newton and Einstein.

Turtle
Turtle Experiment: Portfolio Construction Matters
To explain the significance of portfolio construction, we will start off with a famous quant story. Back in the 1980s, Richard Dennis and William Eckhert made a bet on the importance of nature versus nurture for becoming a great investor. Dennis, a legendary rules based and systematic investor himself turning USD 5,000 into more than 100 million, believed that he could train anyone to become a great investor, while Eckhert disagreed. To settle the bet amongst the two traders, they set up an experiment under the name “turtle trading”, perhaps the most famous experiment in history of finance, in which two separate classes consisting of around 13 people (turtles) learned the art of rule based trading. The main strategy of the program involved buying and selling a diversified set of financial futures contracts on 20 day highs and 20 day lows. The trading result achieved by the turtles varied despite the simplicity of this classic price breakout strategy. This is because a key component is portfolio construction as the rules must be followed 100% of the time, which is really tough when the market is moving against you. Adaptive Alph therefore believes it is important to define portfolio construction and, luckily for us, Rishi Narang does precisely that in his amazing book on quantitative investment, inside the black box. According to Narang, portfolio construction is the master chef blending the signals/spices generated by the alpha (moneymaker), risk and transaction models into an optimal mix in the portfolio. Successful quantitative investors must therefore have a complete understanding of portfolio construction.

Borrowed from Rishi Narang - Portfolio Construction
PS: Alpha model equals the moneymaker
Moneymakers
The goal for a quantitative analyst is to test a profitable hypothesis resulting from careful analysis of mispricing in financial instruments. Adaptive Alph calls the profit generating model the moneymaker, as its objective is to make money. One should consider the moneymaker as the optimist constantly scanning the investment universe for opportunity and as a result, feeding positive buy or sell signals into the portfolio construction part of the portfolio. A hypothesis for the moneymaker is either theory driven or it takes a more statistical, data driven approach. The former is relatively simple to understand involving the classic if “A” happens then “B” should happen reasoning. For example, if the market expects a company to report USD 10 million in earnings, but the company instead ends up reporting USD 100 million, a valid theory is to expect this particular company’s share price to increase. The latter, the data driven approach, is of higher complexity as it requires rigorous training in statistical methods and computer science to extract information emerging from the patterns, often nonlinear, in the data. Unlike the traditional approach, these nonlinear patterns that the quant is capturing do not fly under any theoretical banner, but rather they are patterns which are simply statistically significant. As an investor in a quant fund relying on a data driven approach, the fundamental truth of statistical science is simply something that the investor must believe. If believing in statistics is difficult, then Adaptive Alph does not recommend investing hard earned cash into a fund relying on the data driven approach.
Moneymaker Types and Successful turtles
In general there are six underlying known main factors (data driven models also capture factors difficult to give a name due to their complexity):
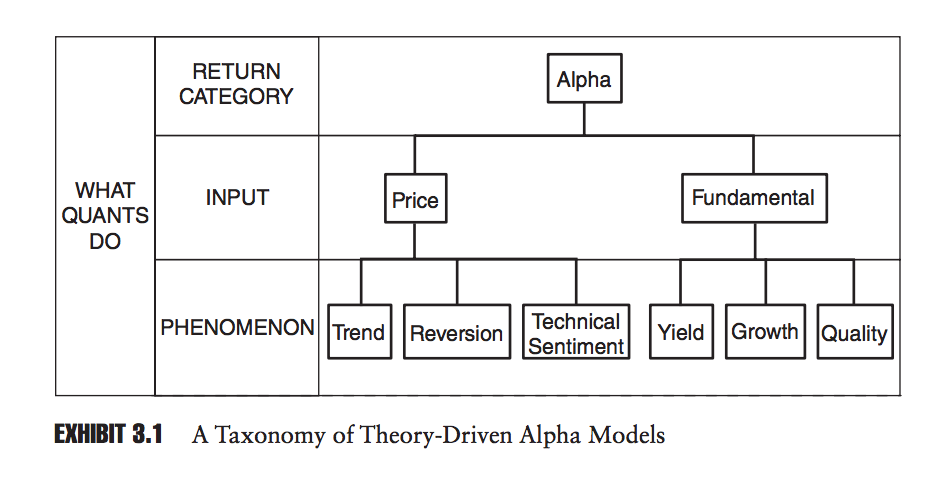
Factors that potentially drives the performance of a quantitative hedge fund
Three of these factors are fundamental factors and three of them are technical factors and all of them are different ways to drive the moneymaker. Fundamentals are factors derived from qualitative and quantitative information contributing to the economic value of a company, currency or asset, while technical factors are derived from human behaviors or sentiments driving the price of a particular asset. In the Dennis and Eckhert example above, the strategy utilize technical factors as the hypothesis is that if the price of a futures contract on, for example, gold breaks above its past 20 day high then the higher price trend is likely to continue and a buy order is therefore issued. If the price continues to increase further buy trades are sent into the portfolio construction optimizer until the price trend turns around and one must have a predefined strategy for exiting the trade. It turns out that Dennis hypothesis about traders being made rather than born was correct as at least six traders, listed below, made millions of dollars dating back to 1986.

- Jerry Parker (Chesapeake)
- Paul Rabar (Rabar)
- Liz Cheval (EMC)
- Howard Seidler (Saxon)
- Tom Shanks (Hawksbill)
- Jim DiMaria (JPD
Jerry Parker - You can listen to him on the weekly podcast Top Traders Unplugged
Risk Models
Generally, the moneymaker is the component that sends the strongest signal into the portfolio construction. The second strongest component is the risk management model, which is the pessimist carefully scrutinizing the moneymaker exposures. In Narang’s book, he separated the risk model from the moneymaking model, but Adaptive Alph believes sophisticated moneymakers tend to have a built in risk management function. Before explaining why risk management is important, we must first define the meaning of risk, which is simply the elimination of unwanted or unintended exposures. Many investors tend to define risk as volatility, dispersion or VaR, but all these measurements fail to capture the embedded risk in the portfolio such as tail risk, event risk, skew and kurtosis. Therefore, Adaptive Alph believes risk management is about careful position selection in regards to both selecting security as well as the position size in that particular security. For example, an exceptional moneymaker in trend following will allocate more risk to instruments with a strong price trend and less risk to those instruments that are whipsawing. This is an intuitive part of a trend following moneymaker. Important to note is that this type of dynamic risk taking is much more difficult to accomplish for a non quantitative trader relying on a more discretionary approach as the reaction time of a computer is multiples quicker at analyzing data than a human brain.

Balancing risk
Transaction Cost Model
Finally, there is the transaction cost model acting as a stingy CFO constantly measuring the trade cost of a signal generated by the moneymaker. The transaction cost model either feeds its own signal into the portfolio construction optimizer or it is a part of the moneymaker. Narang separated out the transaction cost model from the moneymaker to make it easier for the reader to understand its unique role within a portfolio context. Whenever a signal to trade is generated by the moneymaker there are two separate costs. The first cost is the cost of the trade itself and the second cost is the opportunity cost of trading something else. It could also be the case that two models are looking to make trades in the opposite direction and these trades are then netted against each other in some fashion, but this tends to be handled by the portfolio construction part of the portfolio. The job of the transaction cost model is to weight the strength of the signal generated by the moneymaker to its cost. If trade A is expensive with a super strong signal and trade B is cheap with a semi strong signal, the transaction cost model might shift the moneymaker to pick trade B. Important to note is that risk management potentially comes into play here as well and put strength behind trade A if trade A is perceived as less risky by the risk model. Despite the fact that most quant funds trade liquid instruments such as futures or public equities, frequent trading is still expensive and a large chunk of the performance can be subtracted by trading cost.
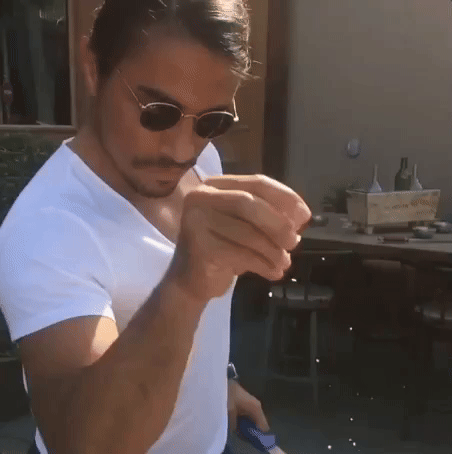
Portfolio construction = Masterchef
The Art of Portfolio Construction
When the moneymaker, risk and transaction models have generated a signal, the portfolio construction makes the ultimate decision on positioning. The decision is done using either a bottom up or top down approach often generating vastly different portfolios. In a bottom up portfolio construction, risk is allocated to moneymakers on a discretionary basis often through the vote of the portfolio’s investment committee. As a result, the moneymaking models act independently from another when generating forecast signals sent to the portfolio optimizer, which preferably includes the built in risk and transaction cost models. The portfolio optimizer is either based on optimization or rules based heuristics, the latter meaning that the ultimate position in each instrument is derived from human trial and error and therefore most often used in a bottom up portfolio construction approach. The advantage of using rules based heuristics is that one can identify precisely which model generates the return thereby allocating more risk to models that seem to perform better. Using heuristics one can also decide upon position limits on both different sectors and individual instruments preventing risk concentration without constraining an optimization process. A bottom up approach is essentially functioning as a fund of funds manager that instead of allocating capital to different fund managers distributes risk to different models. This means that if the hypothetical bottom up portfolio consists of three moneymakers it must be that three separate signals are sent into the portfolio construction. If moneymaker A is short gold with a strong signal and moneymaker B is long with a weak signal, assuming approximately equal weighted risk allocation, the portfolio will most likely short gold unless moneymaker C is strong long or if a rules based heuristic decision prevents the portfolio from further shorting gold as the risk exposure might exceed the system’s limit.
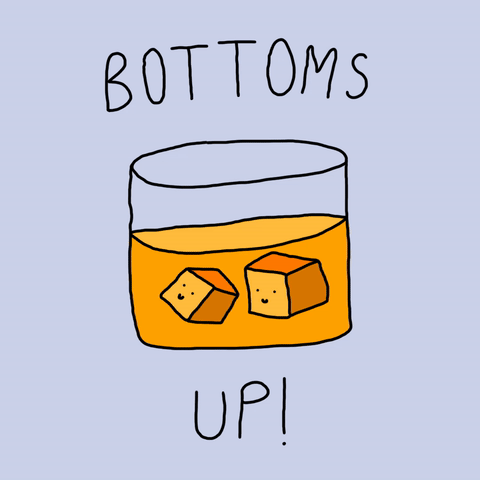
Or Top Down...:)
Top Down versus Bottom Up
Arguably, there are some weaknesses in the bottom up approach and that is why a top down portfolio construction is increasing in popularity with quant managers. The most obvious weakness of the bottom up approach is that the investment committee of a quant portfolio makes risk allocation decisions on a discretionary basis even if the outline guiding the decisions follows a systematic process. What Adaptive Alph means by a systematic process is that if model A performed well since the most recent committee meeting, then model A can at most receive x% higher exposure to avoid personal biases by any investment committee members. Just imagine the skewed allocation impact of endowment bias if one of the committee members created model A. Another possible weakness of the bottom up approach is that the models act independently, which might not be optimal from a risk adjusted portfolio return perspective. It could, for example, be that A and B in the above example are uncorrelated or even negatively correlated, which means that one could allocate more risk to each model for the chance of earning a higher return while lowering risk to ultimately increase risk adjusted return. In a top down approach, both the risk allocation and model independency issue is eliminated as each model in the portfolio is integrated with another. The performance of the quant fund would therefore no longer be attributable to model A, B or C, which is actually seen as a weakness of the top down approach by some investors. Instead, the models respective signal is now combined into one single tradable signal in the portfolio optimizer that is then executed in the market. The marginal contribution of each model to that ultimate signal in the portfolio optimizer is based on past success in identifying patterns in accordance with the portfolio optimization objective function and also its correlation to the signals generated by the other models in the portfolio. As you may have noted, allocating a higher weighting to a model with past success in the portfolio optimization step eliminates the need of an investment committee. This in turn has the benefit of preventing human behavioral biases causing deviation from the stated investment objective of the fund. After deciding upon portfolio construction methodology, the final signal is executed and trades are generated to get market exposure. This is the job of the execution algorithm, which is another blog as it is paramount to the success of a quantitative hedge fund.
Conclusion
No matter if a quant fund uses a theory based bottom up approach or a data driven top down approach, investors in quant funds benefit from the quants ability to take risk dynamically and exploit nonlinear patterns. The top quants are those whom optimally combine data, science and art to create moneymakers, risk models and transaction cost models. The signals generated by those models are then forwarded to the portfolio construction part of the portfolio where they are mixed to create optimal market exposures. The art involved in the outline of the portfolio construction of the quantitative investment portfolio is paramount as it is the ultimate product differentiator for quantitative hedge funds.
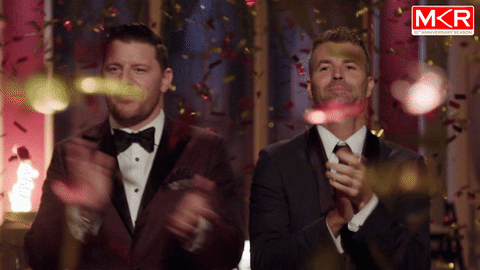
Stay Adaptive!
//Adaptive Alph
Komentarze